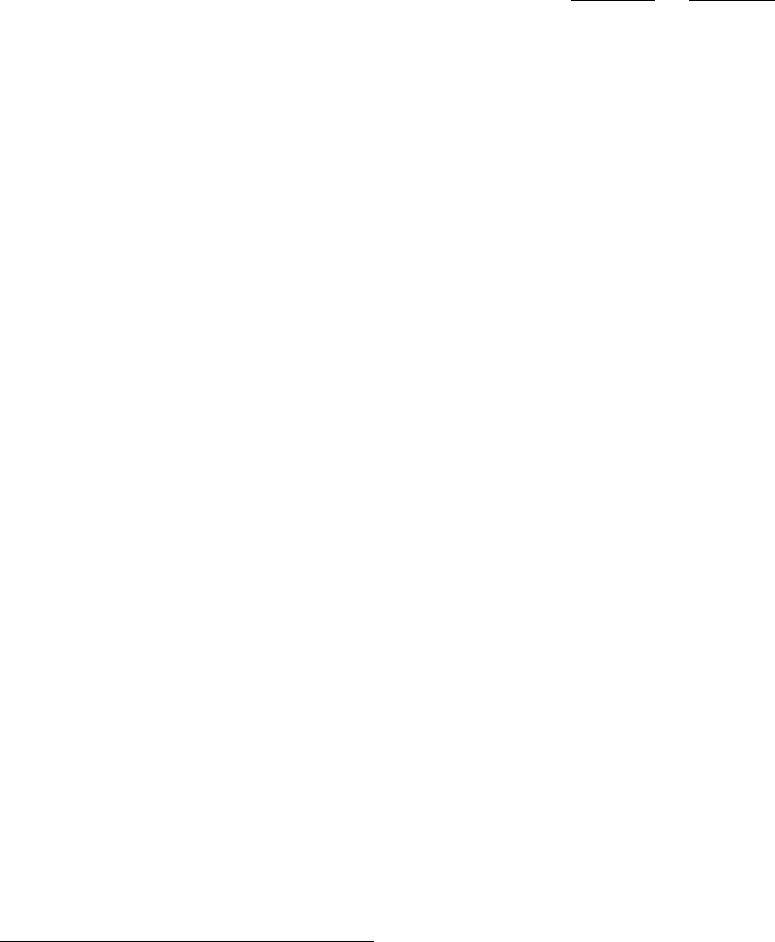
3.3 Patient Utility
For any given service rate µ, a waiting time sensitive patient who seeks admission from the
HCP derives her utility U(λ, µ), where
U(λ, µ) = R − [n(µ) · t + θ · T (λ, µ)] = R −
t
1 − δ(µ)
−
θ
o(µ) − λ
, (7)
in which n(µ) is the number of admissions a patient expects to experience per medical episode
given in (4), T (λ, µ) is the expected total waiting time per medical episode given in (6), R is
the patient’s reward for being cured after the entire episode, t is the patient’s non-pecuniary
disutility associated with each admission, and θ is the imputed cost associated with waiting.
Here, we assume that the waiting time will not cause adverse effects (i.e., worsening patients’
symptoms). This assumption is reasonable for elective surgeries and it is supported by the
empirical evidence established by Hurst and Siciliani (2003).
Knowing the readmission rate and waiting time,
13
each patient will seek admission if and
only if the utility associated with the admission U(λ, µ) ≥ 0.
14
By using the fact that the
utility U(λ, µ) given in (7) is strictly decreasing in λ, we can determine the initial admission
rate
˜
λ(µ) when admissions are endogenously decided by the patients. First, consider the case
when the potential initial admission rate Λ (i.e., the potential arrival rate of newly admitted
patients) is sufficiently large so that U(Λ, µ) < 0 (because U(λ, µ) is strictly decreasing in
λ). In this case, the initial admission rate
˜
λ in equilibrium satisfies U(
˜
λ, µ) = 0, where
˜
λ < Λ, and the balking rate equals (Λ −
˜
λ) (Hassin and Haviv 2003). We shall refer to
this case as the partial coverage scenario. Next, consider the case when the potential initial
admission rate Λ is sufficiently small so that U(Λ, µ) ≥ 0. In this case, all potential patients
will seek admissions so that
˜
λ = Λ. We shall refer to this case as the full coverage scenario.
To avoid repetition and to ease our exposition, we shall present our analysis for the cases
where potential patients are either partially covered or fully covered under both schemes
in the main text, and provide similar analysis for the case where patients are fully covered
under one scheme but are partially covered under the other scheme in the online Appendix
B.
So far, we have established the relationships among the initial admission rate λ, the
readmission rate δ(µ), the patient utility U(., .), the patient’s waiting time per visit W(., .),
13
In many countries, the readmission rate and waiting time are common knowledge. For example, the Aus-
tralian government releases the Australian hospital statistics report at http://www.aihw.gov.au/publication-
detail/?id=60129553174, in which the information regarding the waiting time and the readmission rate for
the elective surgery can be found on page 35 and page 52, respectively.
14
When the imputed disutility associated with each admission or the waiting cost is large enough such
that R−θ ·T (0, µ)−t ·n(µ) < 0 for all µ > 0, the patients’ utility is always negative and therefore, no patient
will seek admission. To avoid this trivial case, hereafter we assume that max
µ>0
{R − θ · T (0, µ) − t · n(µ)} > 0
so that some patients will seek admission in equilibrium.
10