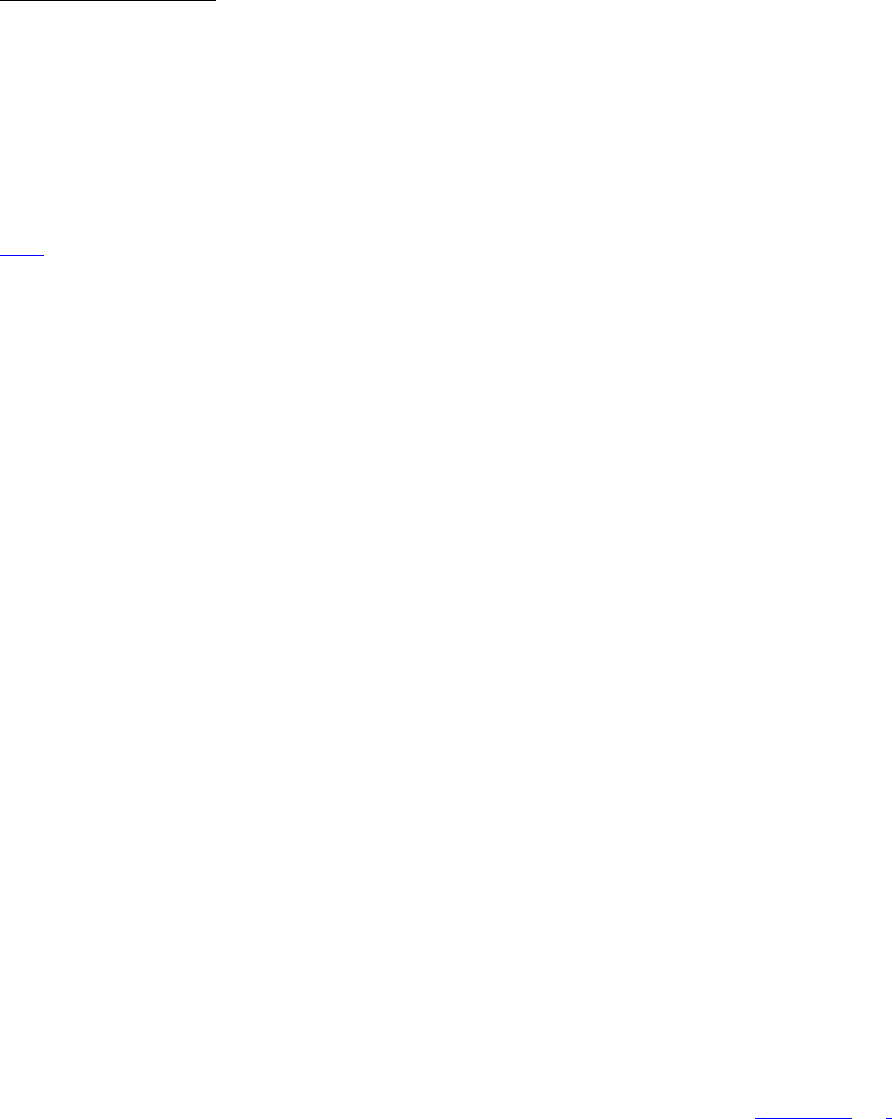
C.2. Soft News Articles:
The HSRATIO for the article about Apple is 0.285, and for the article about Abbott is 0.324.
Apple Plots Its TV Assault
By
Je
ssica E. Vascellaro And
Sam Schechner
December 19, 2011
Apple Inc. is moving forward with its assault on television, following up on the ambitions of its late co-founder, Steve Jobs.
In recent weeks, Apple executives have discussed their vision for the future of TV with media executives at several large companies,
a
ccording to people familiar with the matter.
WSJ's Sam Schechner reports on Apple's plans to build its own television that would feature wireless streaming to access shows, movies and
c
ontent.
Apple is also working on its own television that relies on wireless streaming technology to access shows, movies and other content, according
t
o people briefed on the project.
In the recent meetings with media companies, the Apple executives, including Senior Vice President Eddy Cue, have outlined new ways
A
pple's technology could recognize users across phones, tablets and TVs, people familiar with the talks said.
In at least one meeting, Apple described future television technology that would respond to users' voices and movements, one of the people
sa
id. Such technology, which Apple indicated may take longer than some of its other ideas, might allow users to use their voices to search
for a show or change channels.
Apple is still saying little about what specific software and devices it is working on. The people familiar with the meetings said the Cupertino,
C
alif., company was "vague" and that Apple hasn't made proposals to license shows for any new product offering.
Still, the talks—some of which were made at the request of media companies seeking an update on Apple's plans—suggest that Apple's TV
st
rategy is advancing. The technology company often keeps its products and ideas, close to the vest until as late as possible.
Apple executives have given some specifics in its talks with media companies. The company, for example, has discussed new ways they
c
ould stream media companies' content, allowing a user to watch a video on a TV set, then pick up another device, such as a smartphone, and
keep watching the video on the move, one of the people familiar said.
Another person familiar with the talks said the types of new services Apple and the media companies are discussing could be done with
A
pple's existing technologies, which include its Apple TV set-top box.
Apple's Eddy Cue, seen in October, is involved in the company's TV plans.
Around three months after it started selling a new, $99 version of the set-top box last year, Apple said it had sold more than a million but
h
asn't provided sales figures since.
Apple's uptick in talks with its media partners is part of the company's strategy to change the way consumers watch TV, just as the company
t
ransformed the music and cellphone industries. Mr. Jobs envisioned building a TV that would be controlled by Apple's mobile devices in
order to be easier to use and more personalized, according to people familiar with the matter.
The company has worked on prototypes for years. Before his death in October, Mr. Jobs told biographer Walter Isaacson that he had "finally
c
racked it," according to Mr. Isaacson's book.
The TV device Apple is working on would use a version of Apple's wireless-streaming technology AirPlay to allow users to control it from
i
Phones and iPads, according to people briefed on the matter. When the company plans to start selling such a device and whether it would
receive traditional broadcast or cable signals remains unclear, said these people, who say Apple may change its plans.
The technology could allow users to stream video from mobile devices to their televisions, without a set-top box. That process is already
p
ossible through its Apple TV set-top box, but it is cumbersome and some media companies, such as Time Warner Inc. 's cable channel
HBO, prevent their apps from using the technology because they want closer control of how and where their content appears. An HBO
spokesman says it hopes to use AirPlay once it is comfortable with the antipiracy protection.
Apple has worked on technologies for integrating DVR storage and iCloud, its online syncing and storage service, into the device, according
t
o a person briefed on the matter. Such technologies could allow users to watch shows they have saved or purchased on two different devices,
like a TV and a computer, without having to buy or record the shows twice.
Other media outlets have reported that Apple is developing a TV. An Apple spokesman declined to comment.
43