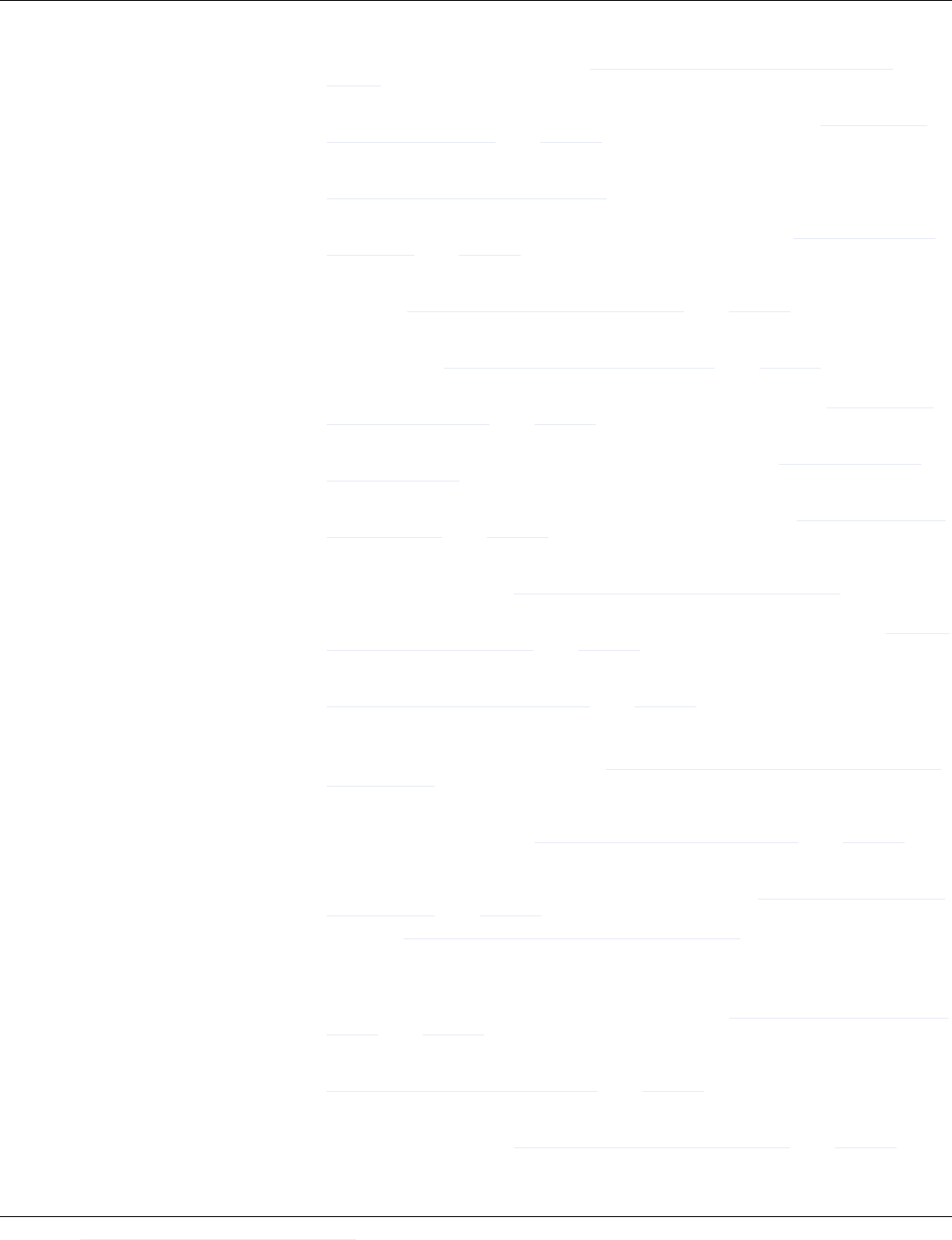
6. Sheth TN, Choudhry NK, Bowes M, Detsky AS. The relation of conjunctival pallor to the presence of
anemia. J Gen Intern Med 1997; 12:102–6. https://doi.org/10.1046/j.1525-1497.1997.00014.x PMID:
9051559
7. Collings S, Thompson O, Hirst E, Goossens L, George A, Weinkove R. Non-invasive detection of anae-
mia using digital photographs of the conjunctiva. PLOS ONE 2016; 11:e0153286. https://doi.org/10.
1371/journal.pone.0153286 PMID: 27070544
8. Appiahene P, Arthur EJ, Korankye S, Afrifa S, Asare JW, Donkoh ET. Detection of anemia using con-
junctiva images: A smartphone application approach. Med Nov Technol Devices 2023; 18:100237.
https://doi.org/10.1016/j.medntd.2023.100237
9. Park SM, Visbal-Onufrak MA, Haque MM, Were MC, Naanyu V, Hasan MK, et al. mHealth spectros-
copy of blood hemoglobin with spectral super-resolution. Optica 2020; 7:563. https://doi.org/10.1364/
optica.390409 PMID: 33365364
10. Dimauro G, Griseta ME, Camporeale MG, Clemente F, Guarini A, Maglietta R. An intelligent non-inva-
sive system for automated diagnosis of anemia exploiting a novel dataset. Artif Intell Med 2023;
136:102477. https://doi.org/10.1016/j.artmed.2022.102477 PMID: 36710064
11. Perez-Plazola MS, Tyburski EA, Smart LR, Howard TA, Pfeiffer A, Ware RE, et al. AnemoCheck-LRS:
an optimized, color-based point-of-care test to identify severe anemia in limited-resource settings. BMC
Med 2020; 18:337. https://doi.org/10.1186/s12916-020-01793-6 PMID: 33190639
12. Haggenmu¨ller V, Bogler L, Weber A-C, Kumar A, Ba
¨
rnighausen T, Danquah I, et al. Smartphone-based
point-of-care anemia screening in rural Bihar in India. Commun Med 2023; 3:1–10. https://doi.org/10.
1038/s43856-023-00267-z PMID: 36949164
13. Bauskar S, Jain P, Gyanchandani M. A noninvasive computerized technique to detect anemia using
images of eye conjunctiva. Pattern Recognit Image Anal 2019; 29:438–46. https://doi.org/10.1134/
S1054661819030027
14. Chen Y-M, Miaou S-G, Bian H. Examining palpebral conjunctiva for anemia assessment with image
processing methods. Comput Methods Programs Biomed 2016; 137:125–35. https://doi.org/10.1016/j.
cmpb.2016.08.025 PMID: 28110719
15. Rivero-Palacio M, Alfonso-Morales W, Caicedo-Bravo E. Mobile application for anemia detection
through ocular conjunctiva images. 2021 IEEE Colomb. Conf. Appl. Comput. Intell. ColCACI, Cali,
Colombia: IEEE; 2021, p. 1–6. https://doi.org/10.1109/ColCACI52978.2021.9469593.
16. Mannino RG, Myers DR, Tyburski EA, Caruso C, Boudreaux J, Leong T, et al. Smartphone app for non-
invasive detection of anemia using only patient-sourced photos. Nat Commun 2018; 9:4924. https://doi.
org/10.1038/s41467-018-07262-2 PMID: 30514831
17. Korevaar DA, Cohen JF, Reitsma JB, Bruns DE, Gatsonis CA, Glasziou PP, et al. Updating standards
for reporting diagnostic accuracy: the development of STARD 2015. Res Integr Peer Rev 2016; 1:7.
https://doi.org/10.1186/s41073-016-0014-7 PMID: 29451535
18. Badireddy M, Baradhi KM. Chronic Anemia. StatPearls, Treasure Island (FL): StatPearls Publishing;
2023.
19. Transfusion thresholds adult—UpToDate n.d. https://www.uptodate.com/contents/image?imageKey=
HEME%2F93934 (accessed June 29, 2023).
20. Moretti K, Marque
´
s CG, Garbern S, Mbanjumucyo G, Uwamahoro C, Beaudoin FL, et al. Transfusion,
mortality and hemoglobin level: Associations among emergency department patients in Kigali, Rwanda.
Afr J Emerg Med 2020; 10:68–73. https://doi.org/10.1016/j.afjem.2020.01.004 PMID: 32612911
21. Stevens GA, Paciorek CJ, Flores-Urrutia MC, Borghi E, Namaste S, Wirth JP, et al. National, regional,
and global estimates of anaemia by severity in women and children for 2000–19: a pooled analysis of
population-representative data. Lancet Glob Health 2022; 10:e627–39. https://doi.org/10.1016/S2214-
109X(22)00084-5 PMID: 35427520
22. Anemia n.d. https://www.hematology.org/education/patients/anemia (accessed July 3, 2023).
23. America Counts Staff. RHODE ISLAND: 2020 Census. U S Census Bur 2021.
24. Safiri S, Kolahi A-A, Noori M, Nejadghaderi SA, Karamzad N, Bragazzi NL, et al. Burden of anemia and
its underlying causes in 204 countries and territories, 1990–2019: results from the Global Burden of Dis-
ease Study 2019. J Hematol OncolJ Hematol Oncol 2021; 14:185. https://doi.org/10.1186/s13045-021-
01202-2 PMID: 34736513
25. Edwards P, Zhang C, Zhang B, Hong X, Nagarajan VK, Yu B, et al. Smartphone based optical spec-
trometer for diffusive reflectance spectroscopic measurement of hemoglobin. Sci Rep 2017; 7:12224.
https://doi.org/10.1038/s41598-017-12482-5 PMID: 28939898
26. Wemyss TA, Nixon-Hill M, Outlaw F, Karsa A, Meek J, Enweronu-Laryea C, et al. Feasibility of smart-
phone colorimetry of the face as an anaemia screening tool for infants and young children in Ghana.
PloS One 2023; 18:e0281736. https://doi.org/10.1371/journal.pone.0281736 PMID: 36867642
Anemia prediction using a smartphone camera
PLOS ONE | https://doi.org/10.1371/journal.pone.0302883 May 13, 2024 12 / 13