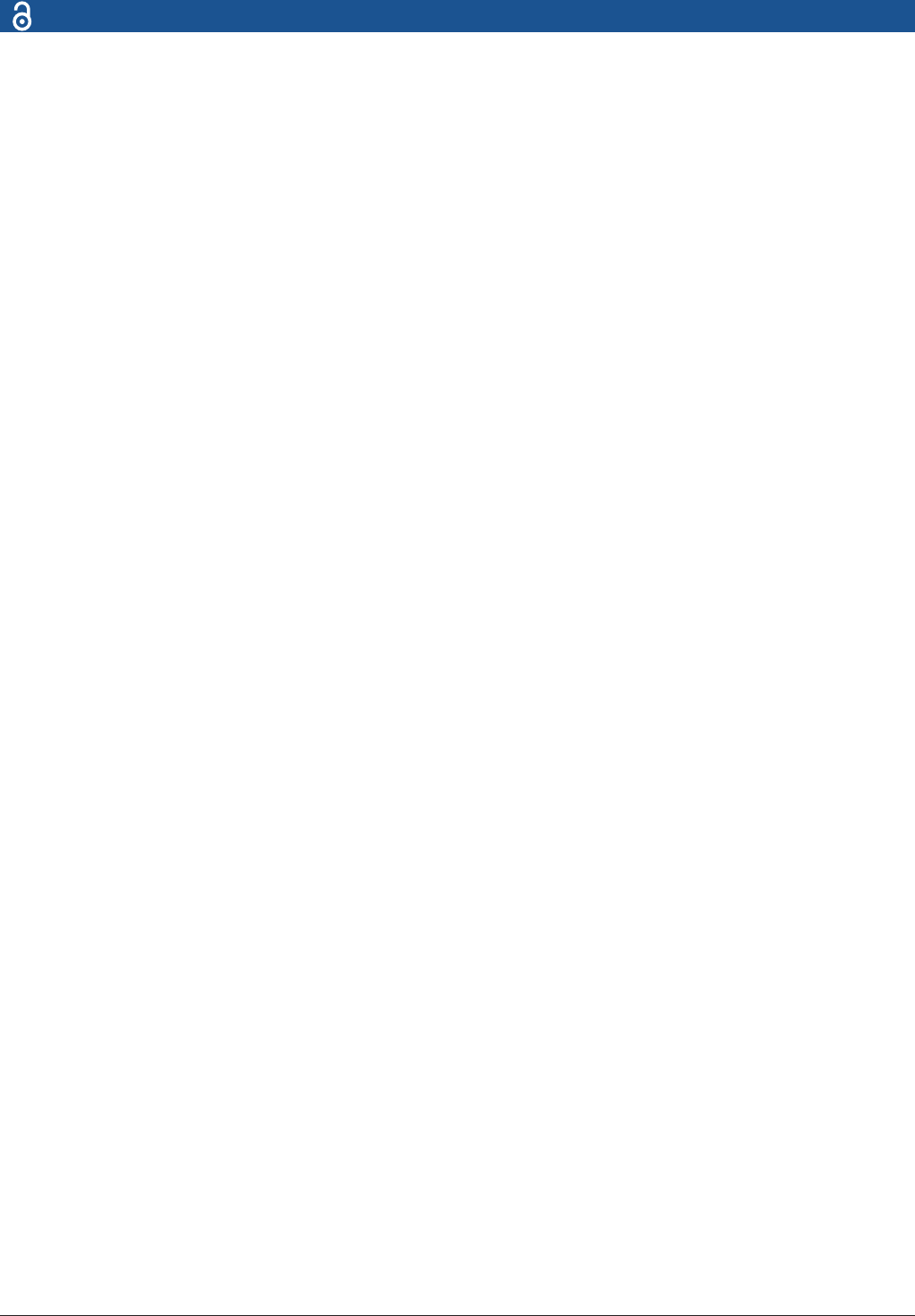
AguasR, etal. BMJ Global Health 2020;5:e003126. doi:10.1136/bmjgh-2020-003126
9
BMJ Global Health
5 Davies NG, Kucharski AJ, Eggo RM, etal. Effects of non-
pharmaceutical interventions on COVID-19 cases, deaths, and
demand for hospital services in the UK: a modelling study. Lancet
Public Health 2020;5:e375–85.
6 Cowling BJ, Ali ST, Ng TWY, etal. Impact assessment of non-
pharmaceutical interventions against coronavirus disease 2019 and
inuenza in Hong Kong: an observational study. Lancet Public Health
2020;5:e279–88.
7 Habib H. Has Sweden's controversial covid-19 strategy been
successful? BMJ 2020;369:m2376.
8 Rhodes T, Lancaster K, Lees S, etal. Modelling the pandemic:
attuning models to their contexts. BMJ Glob Health 2020;5:e002914.
9 Freebairn L, Rychetnik L, Atkinson J- A, etal. Knowledge
mobilisation for policy development: implementing systems
approaches through participatory dynamic simulation modelling.
Health Res Policy Syst 2017;15:83.
10 Mansnerus E. Using model- based evidence in the governance of
pandemics. Sociol Health Illn 2013;35:280–91.
11 Leach M, Scoones I. The social and political lives of zoonotic
disease models: narratives, science and policy. Soc Sci Med
2013;88:10–17.
12 Zhou F, Yu T, Du R, etal. Clinical course and risk factors for mortality
of adult inpatients with COVID-19 in Wuhan, China: a retrospective
cohort study. Lancet 2020;395:1054–62.
13 CDC COVID-19 Response Team. Severe Outcomes Among Patients
with Coronavirus Disease 2019 (COVID-19) - United States, February
12- March 16, 2020. MMWR Morb Mortal Wkly Rep 2020;69:343–6.
14 nychealth / coronavirus- data, 2020. Available: https:// github. com/
nychealth/ coronavirus- data/ blob/ master/ by- age. csv
15 Onder G, Rezza G, Brusaferro S. Case- Fatality rate and
characteristics of patients dying in relation to COVID-19 in Italy.
JAMA 2020;323:1775-1776.
16 Oke J, Heneghan C. Global Covid-19 case fatality rates, 2020.
Available: https://www. cebm. net/ covid- 19/ global- covid- 19- case-
fatality- rates/
17 Wu Z, McGoogan JM. Characteristics of and Important Lessons
From the Coronavirus Disease 2019 (COVID-19) Outbreak in
China: Summary of a Report of 72 314 Cases From the Chinese
Center for Disease Control and Prevention. JAMA 2020;323:1239-
1242.
18 Bi Q, Wu Y, Mei S, etal. Epidemiology and transmission of
COVID-19 in Shenzhen China: analysis of 391 cases and 1286 of
their close contacts. medRxiv 2020.
19 Lauer SA, Grantz KH, Bi Q, etal. The incubation period of
coronavirus disease 2019 (COVID-19) from publicly reported
conrmed cases: estimation and application. Ann Intern Med
2020;172:577–82.
20 Wei WE, Li Z, Chiew CJ, etal. Presymptomatic Transmission of
SARS- CoV-2 - Singapore, January 23- March 16, 2020. MMWR Morb
Mortal Wkly Rep 2020;69:411–5.
21 Arons MM, Hateld KM, Reddy SC, etal. Presymptomatic SARS-
CoV-2 infections and transmission in a skilled nursing facility. N Engl
J Med 2020;382:2081–90.
22 Tong Z- D, Tang A, Li K- F, etal. Potential presymptomatic
transmission of SARS- CoV-2, Zhejiang Province, China, 2020.
Emerg Infect Dis 2020;26:1052–4.
23 To KK- W, Tsang OT- Y, Leung W- S, etal. Temporal proles of viral
load in posterior oropharyngeal saliva samples and serum antibody
responses during infection by SARS- CoV-2: an observational cohort
study. Lancet Infect Dis 2020;20:565–74.
24 He X, Lau EHY, Wu P, etal. Temporal dynamics in viral shedding and
transmissibility of COVID-19. Nat Med 2020;26:672–5.
25 Li P, Fu J- B, Li K- F, etal. Transmission of COVID-19 in the terminal
stages of the incubation period: a familial cluster. Int J Infect Dis
2020;96:452–3.
26 Nishiura H, Linton NM, Akhmetzhanov AR. Serial interval of novel
coronavirus (COVID-19) infections. Int J Infect Dis 2020;93:284–6.
27 Du Z, Xu X, Wu Y, etal. Serial interval of COVID-19 among publicly
reported conrmed cases. Emerg Infect Dis 2020;26:1341–3.
28 Wang D, Hu B, Hu C, etal. Clinical characteristics of 138
hospitalized patients with 2019 novel coronavirus- infected
pneumonia in Wuhan, China. JAMA 2020;323:1061.
29 Goyal P, Choi JJ, Pinheiro LC, etal. Clinical characteristics of
Covid-19 in New York City. N Engl J Med 2020;382:2372–4.
30 Verity R, Okell LC, Dorigatti I, etal. Estimates of the severity of
coronavirus disease 2019: a model- based analysis. Lancet Infect Dis
2020;20:669–77.
31 Russell TW, Hellewell J, Jarvis CI, etal. Estimating the infection and
case fatality ratio for coronavirus disease (COVID-19) using age-
adjusted data from the outbreak on the diamond Princess cruise
SHIP, February 2020. Euro Surveill 2020;25.
32 Mizumoto K, Chowell G. Estimating risk for death from coronavirus
disease, China, January- February 2020. Emerg Infect Dis
2020;26:1251–6.
33 Winter AK, Hegde ST. The important role of serology for COVID-19
control. Lancet Infect Dis 2020;20:758–9.
34 Xiang F, Wang X, He X, etal. Antibody detection and dynamic
characteristics in patients with COVID-19. Clin Infect Dis 2020.
35 Yong SEF, Anderson DE, Wei WE, etal. Connecting clusters of
COVID-19: an epidemiological and serological investigation. Lancet
Infect Dis 2020;20:809–15.
36 Yang J, Zheng Y, Gou X, etal. Prevalence of comorbidities and its
effects in patients infected with SARS- CoV-2: a systematic review
and meta- analysis. Int J Infect Dis 2020;94:91–5.
37 Halpin DMG, Faner R, Sibila O, etal. Do chronic respiratory diseases
or their treatment affect the risk of SARS- CoV-2 infection? Lancet
Respir Med 2020;8:436–8.
38 Emanuel EJ, Persad G, Upshur R, etal. Fair allocation of scarce
medical resources in the time of Covid-19. N Engl J Med Overseas
Ed 2020;382:2049–55.
39 Wang B, Li R, Lu Z, etal. Does comorbidity increase the risk of
patients with COVID-19: evidence from meta- analysis. Aging
2020;12:6049–57.
40 MacIntyre CR, Heslop DJ. Public health, health systems and
palliation planning for COVID-19 on an exponential timeline. Med J
Aust 2020.
41 Touboul- Lundgren P, Jensen S, Drai J, etal. Identication of cultural
determinants of antibiotic use cited in primary care in Europe: a
mixed research synthesis study of integrated design "Culture is all
around us". BMC Public Health 2015;15:908.
42 Hale T, Angrist N, Kira B. “Variation in Government Responses to
COVID-19” Version 5.0. Blavatnik School of Government Working
Paper, 2020.
43 Guan Y, Deng H, Zhou X. Understanding the impact of the COVID-19
pandemic on career development: insights from cultural psychology.
J Vocat Behav 2020;119:103438.
44 Messner W. The institutional and cultural context of cross- national
variation in COVID-19 outbreaks. medRxiv 2020.
45 Shim E, Tariq A, Choi W, etal. Transmission potential and severity of
COVID-19 in South Korea. Int J Infect Dis 2020;93:339–44.
46 Karamouzian M, Madani N. COVID-19 response in the middle East
and North Africa: challenges and paths forward. Lancet Glob Health
2020;8:e886–7.
47 Jarvis CI, Van Zandvoort K, Gimma A, etal. Quantifying the impact
of physical distance measures on the transmission of COVID-19 in
the UK. BMC Med 2020;18:124.
48 Prem K, Liu Y, Russell TW, etal. The effect of control strategies
to reduce social mixing on outcomes of the COVID-19 epidemic
in Wuhan, China: a modelling study. Lancet Public Health
2020;5:e261–70.
49 Tian H, Liu Y, Li Y, etal. An investigation of transmission control
measures during the rst 50 days of the COVID-19 epidemic in
China. Science 2020;368:638–42.
50 Kucharski AJ, Klepac P, Conlan AJK, etal. Effectiveness of isolation,
testing, contact tracing, and physical distancing on reducing
transmission of SARS- CoV-2 in different settings: a mathematical
modelling study. Lancet Infect Dis 2020;20:1151–60.
51 Salje H, Tran Kiem C, Lefrancq N, etal. Estimating the burden of
SARS- CoV-2 in France. Science 2020;369:208–11.
52 Di Domenico L, Pullano G, Sabbatini CE, etal. Expected impact of
lockdown in Île- de- France and possible exit strategies. medRxiv 2020.
53 Shretta R. The economic impact of COVID-19, 2020. Available:
https://www. tropicalmedicine. ox. ac. uk/ news/ the- economic- impact-
of- covid- 19
54 Freebairn L, Atkinson J- A, Kelly PM, etal. Decision makers'
experience of participatory dynamic simulation modelling: methods
for public health policy. BMC Med Inform Decis Mak 2018;18:131.
55 Pan- Ngum W, Kinyanjui T, Kiti M, etal. Predicting the relative
impacts of maternal and neonatal respiratory syncytial virus (RSV)
vaccine target product proles: a consensus modelling approach.
Vaccine 2017;35:403–9.
56 Cornell COVID caseload calculator with capacity and ventilators
(C5V Online*), 2020. Available: https:// covid19. sjsu. edu/ C5V/ default/
user/ login?_ next=/ C5V/ default/ index
on August 3, 2024 by guest. Protected by copyright.http://gh.bmj.com/BMJ Glob Health: first published as 10.1136/bmjgh-2020-003126 on 23 December 2020. Downloaded from