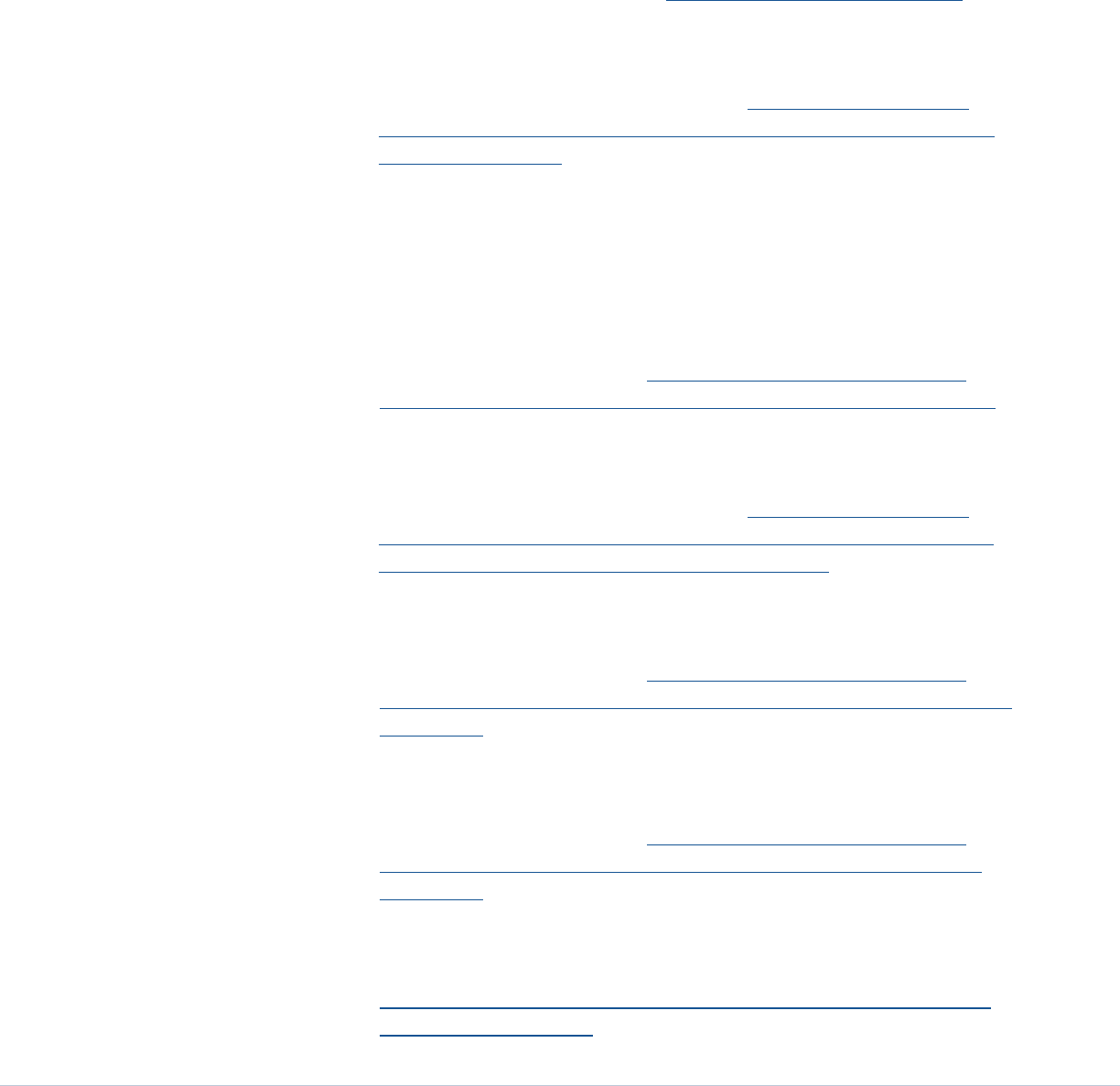
REFERENCES
Bartik, A. W., Bertrand, M., Lin, F., Rothstein, J., & Unrath, M. (2020). Measuring
the Labor Market at the Onset of the COVID-19 Crisis. Brookings Papers on
Economic Activity, 2020(2), 239–268. https://doi.org/10.1353/eca.2020.0010
Bell, A., Hedin, T., Mannino, P., Moghadam, R., Schnoor, G., & Von Wachter,
T. (2021). Re-employment, Recall, and Industry Transitions During the COVID-
19 Pandemic (UI Report). California Policy Lab. https://www.capolicylab.org/
wp-content/uploads/2021/12/Dec-2021-Analysis-of-Unemployment-Insurance-
Claims-in-California.pdf
Bell, A., Hedin, T., Mannino, P., Moghadam, R., Schnoor, G., & Von Wachter, T.
(2022). Disparities in Access to Unemployment Insurance During the COVID-19
Pandemic: Lessons from U.S. and California Claims Data. California Policy Lab.
Bell, A., Hedin, T., Moghadam, R., Schnoor, G., & Von Wachter, T. (2021a).
Why Extended UI Benets were Turned O Prematurely for Workers in 33 States
(UI Report). California Policy Lab. https://www.capolicylab.org/wp-content/
uploads/2021/04/Why-Extended-UI-Benets-Turned-O-Early-in-33-States.pdf
Bell, A., Hedin, T., Moghadam, R., Schnoor, G., & Von Wachter, T. (2021b).
An Analysis of Unemployment Insurance Claims in California During the COVID-
19 Pandemic (UI Report). California Policy Lab. https://www.capolicylab.org/
wp-content/uploads/2021/12/June-30th-Analysis-of-Unemployment-Insurance-
Claims-in-California-During-the-COVID-19-Pandemic.pdf
Bell, A., Hedin, T., Schnoor, G., & Von Wachter, T. (2020a). An Analysis of
Unemployment Insurance Claims in California During the COVID-19 Pandemic
(UI Report). California Policy Lab. https://www.capolicylab.org/wp-content/
uploads/2020/10/Sept-15th-Analysis-of-UI-Claims-in-CA-During-the-COVID-19-
Pandemic.pdf
Bell, A., Hedin, T., Schnoor, G., & Von Wachter, T. (2020b). An Analysis of
Unemployment Insurance Claims in California During the COVID-19 Pandemic
(UI Report). California Policy Lab. https://www.capolicylab.org/wp-content/
uploads/2021/02/Dec-21st-Analysis-of-CA-UI-Claims-during-the-COVID-19-
Pandemic.pdf
Bell, A., Hedin, T., Schnoor, G., & von Wachter, T. (2021). As Crisis Continues, More
Unemployed Californians are Receiving UI Benets (UI Report). California Policy Lab.
https://www.capolicylab.org/wp-content/uploads/2021/02/CPL-Data-Point-on-
Recipiency-in-California.pdf
52 10 KEY INSIGHTS FROM THE COVID-19 PANDEMICcapolicylab.org