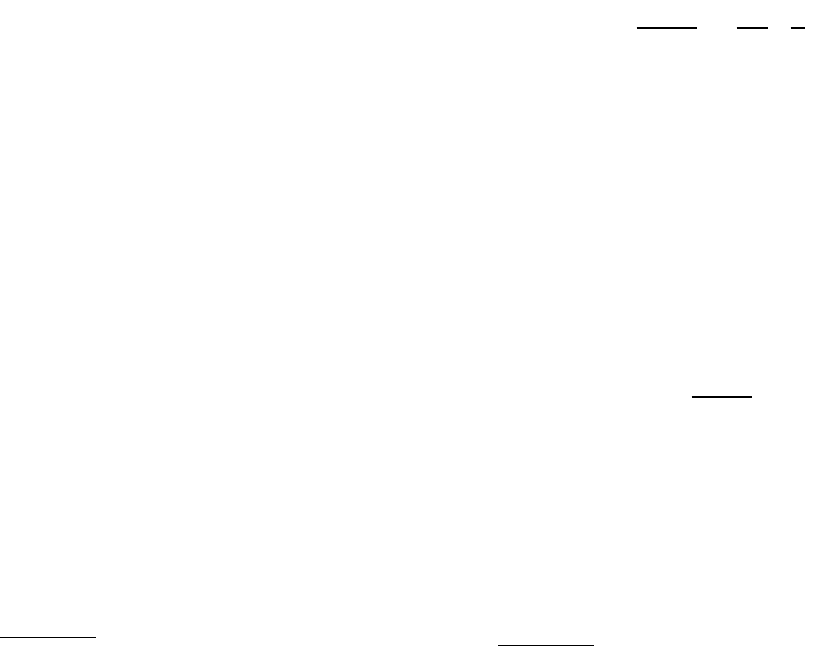
10 e Center for Hospitality Research • Cornell University
e results of review scores move in the other direc-
tion. Using Travelocity’s 5-point score for user reviews, the
Review Score odds ratio means that increasing one point
increases the odds of being selected by 14.2 percent Using
the example of a hotel that goes from a review score of, say,
3.3 to one of 4.3, that property has increased its odds of be-
ing selected by 1.142 times the odds when its review score
was 3.3. A similar result is found for Number of reviews.
e 1.002 indicates that for each new review a hotel adds, it
increases its odds of being selected increase by 1.002 or 0.2
percent.
It is a little harder to interpret the odds ratio of price in
this equation because this variable is actually relative price.
Price is perhaps best understood by combining the eects
of price and review scores. Say that the average price of all
competing hotels (those with the same star rating) that ap-
pear on the list page with our hotel was $100 and our hotel’s
price was $100. If we were to increase our review score from
3.3 to 4.3 we could increase our price about 11.2 percent and
maintain the same probability of being selected. e posi-
tive eect of the review score change on the odds of being
chosen would oset the presumably negative odds caused by
a price increase.
Impact on Hotel Performance
Taking the analysis one step further, I analyze the eect of
social presence on overall hotel performance, again using
matched samples of performance data and online reputation
data. Performance data are monthly revenue, demand, and
supply data from STR for 2½ years (January 2010 through
June 2012) for each subject property. I also compared each
hotel with its specied list of competitors, to gain a sense of
relative performance in an eort to control for seasonality.
I have these data for 11 major markets (6 European and 5
North American cities).
8
I use ReviewPro’s Global Review Index (GRI) for the
subject hotels as well as each hotel within its STR listed com-
petitive set as a measure of online reputation. ReviewPro
aggregates hundreds of millions of social media mentions,
in over 35 languages, from Online Travel Agencies (OTAs),
review websites and social media platforms. eir GRI is
an aggregate online reputation score for an individual hotel,
group of hotels, or chain. It is based on scores given by re-
viewers on major online review sites and online travel agen-
cies (OTAs). e GRI is calculated by analyzing quantitative
scores on these sites, using a proprietary algorithm.
9
With this matched data set we look at the impact of GRI
on three typical industry metrics: ADR (average daily rate),
8
Berlin, Chicago, London, Madrid, Miami, Milan, Los Angeles, New
York, Prague, Rome, and San Francisco.
9
More details on the Global Review Index can be found at www.review-
pro.com/product/global-review-index.
occupancy, and RevPAR (revenue per available room). In all
cases, I am using an index. us the measurements are the
eect of GRI against a hotel’s pricing power as measured by
its ADR Index, which is a hotel’s average daily rate divided
by the average of its competitors’ ADRs; the GRI’s impact
upon demand as measured by the occupancy index, and on
overall performance as measured by GRI upon a RevPAR
index. Likewise, the GRI Index is calculated as the subject
hotel’s GRI divided by the average GRI of its competitive set
hotels. is GRI Index is the independent variable. Similar
to many marketing actions
(e.g., advertising and pricing)
we can anticipate decreasing marginal returns.
10
at is, as
the GRI score increases the additional impact upon per-
formance decreases. To incorporate decreasing marginal
returns, I use a multiplicative model of impact oen referred
to as a constant elasticity model. Using price and demand as
an example I illustrate this approach as follows.
Price elasticity of demand is dened as the percentage
change in demand for a given percentage change in price. So,
for example, if price increased by1 percent, and as a result
demand fell 2 percent, then elasticity is -2 (-2%/1%). Price
elasticity (ε) can be expressed as:
where ε is the price elasticity, P is the price, and Q is the
quantity demanded.
If we propose decreasing marginal returns, a demand
model might look like Q=aPb. If we take the natural loga-
rithm (the inverse of Euler’s number) of each side of this
equation we get a log-linear demand model of the following
form:
lnQ = a + b·lnP
where Q and P are dened as before, and a and b are pa-
rameters to be estimated. e log-linear demand function
implies that the price elasticity of demand is constant:
Using data from over 50,000 monthly observations
from the eleven global cities, we can look at the impact of
GRI upon performance in this log linear framework where
I model the impact of ln(GRI Index) upon ln(ADR Index),
ln(Occupancy Index), and ln(RevPAR Index), using three
log linear models. Exhibit 5 summarizes the elasticities
for GRI upon these three performance metrics. e table
indicates a stronger impact of GRI upon pricing power
10
For an example of the diminishing marginal returns principle, see:
Joseph omas, “Linear Programming Models for Production-Adver-
tising Decisions,” Management Science, Vol. 17, No. 8 (April 1971), pp.
B-474–B-484.
ε =
dlnQ
=
%∆Q
%∆P
∂Q
∂P
ε =
P
Q
dlnP
b
=